Ai Vs Rpa
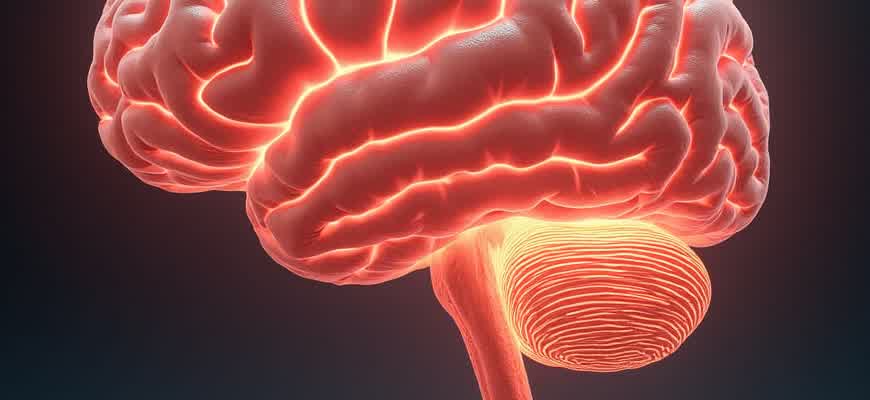
Artificial Intelligence (AI) and Robotic Process Automation (RPA) are often viewed as complementary technologies, but they serve different purposes and have unique capabilities in the business process landscape.
AI is designed to simulate human intelligence by processing large amounts of data, learning from it, and making decisions based on this knowledge. It can handle tasks that require cognitive abilities such as natural language processing, pattern recognition, and problem-solving. On the other hand, RPA automates repetitive, rule-based tasks, performing them faster and with fewer errors than humans.
Key differences between the two technologies are summarized in the table below:
Feature | AI | RPA |
---|---|---|
Purpose | Decision-making, learning from data | Automating routine, repetitive tasks |
Complexity | Can handle complex, dynamic scenarios | Operates in a structured environment |
Flexibility | Highly flexible, adapts to new situations | Limited flexibility, works within predefined rules |
AI enables systems to make judgments and improve over time, while RPA is focused on executing well-defined tasks without deviation from established processes.
The strengths of RPA lie in its ability to automate processes at scale with minimal intervention, which is particularly useful in environments where consistency and efficiency are paramount. AI, however, offers more profound advantages by enabling machines to understand context, process unstructured data, and improve their performance through machine learning.
AI vs RPA: Understanding the Key Differences and Use Cases
Artificial Intelligence (AI) and Robotic Process Automation (RPA) are two transformative technologies that are revolutionizing industries globally. While both contribute to automation and efficiency, they serve different purposes and operate in distinct ways. AI focuses on mimicking human intelligence and decision-making processes, whereas RPA automates repetitive tasks based on pre-set rules. Understanding their differences is crucial for businesses looking to adopt the right technology for their needs.
While RPA is best suited for automating high-volume, rule-based tasks, AI enables machines to learn from data and adapt their behavior over time. This leads to the ability to handle complex, unpredictable processes. Below, we explore the key distinctions and typical use cases of AI and RPA.
Key Differences Between AI and RPA
Feature | AI | RPA |
---|---|---|
Functionality | Simulates human thinking and decision-making processes | Automates repetitive, rule-based tasks |
Adaptability | Can learn and adapt from data | Operates based on predefined scripts and workflows |
Complexity | Handles unstructured and complex data | Focuses on structured, simple processes |
Integration | Requires deeper integration with business processes | Can integrate quickly with existing systems and software |
Use Cases for AI and RPA
- AI: Used in areas requiring decision-making, natural language processing, predictive analytics, and machine learning. Examples include fraud detection, customer support chatbots, and personalized recommendations.
- RPA: Ideal for automating repetitive, high-volume tasks like data entry, invoice processing, and payroll management.
Important Considerations
AI is best suited for processes that require learning, decision-making, and adapting to changing environments. In contrast, RPA excels in automating well-defined, repetitive tasks that follow strict rules and workflows.
How AI Enhances Business Decision-Making Compared to RPA
Artificial Intelligence (AI) has a more profound impact on business decision-making than Robotic Process Automation (RPA). While RPA focuses on automating repetitive tasks, AI takes decision-making to a deeper level by analyzing large datasets, predicting trends, and recommending optimal actions. This allows businesses to make more informed, data-driven decisions that lead to better outcomes in real-time scenarios.
AI's ability to process and interpret vast amounts of unstructured data–such as customer feedback, social media sentiment, and market conditions–makes it an indispensable tool for strategic planning. RPA, on the other hand, is limited to rule-based automation, meaning it cannot make decisions based on context or adapt to changes in data patterns.
Key Differences in Decision-Making Capabilities
- Data Processing: AI excels at analyzing complex data, while RPA is only capable of executing predefined tasks.
- Adaptability: AI adapts and learns from new data, enhancing decision-making over time. RPA relies on fixed rules and cannot adjust to changes dynamically.
- Predictive Power: AI can predict trends and behaviors based on historical data, whereas RPA does not have predictive capabilities.
How AI Enhances Decision-Making
- Real-Time Analysis: AI systems can analyze live data streams, allowing businesses to make decisions quickly as circumstances evolve.
- Advanced Recommendations: AI provides insights and suggestions based on patterns and correlations that might not be immediately apparent to human analysts.
- Risk Assessment: AI systems assess potential risks and can propose strategies to mitigate them before they escalate.
AI is not just about automating tasks; it’s about augmenting decision-making through data-driven insights and continuous learning.
Comparison Table: AI vs RPA in Decision-Making
Feature | AI | RPA |
---|---|---|
Data Analysis | Complex and unstructured data | Structured, rule-based data |
Adaptability | Can learn and evolve | Fixed and predefined rules |
Predictive Capabilities | Yes | No |
Risk Mitigation | Identifies and proposes solutions | No |
Choosing the Right Solution for Customer Support Automation: AI vs RPA
When considering the automation of customer support processes, organizations often face a critical decision between two powerful technologies: Artificial Intelligence (AI) and Robotic Process Automation (RPA). Both options offer unique benefits, but understanding their differences is key to selecting the most effective approach for a specific business need. AI excels in tasks that require decision-making, context understanding, and adaptation to dynamic customer queries, while RPA is better suited for repetitive, rule-based tasks that involve structured data.
The right choice depends on the complexity of the support process. AI can enhance customer experience by enabling sophisticated interactions through chatbots, virtual assistants, or voice recognition systems. On the other hand, RPA can streamline operations by automating manual, repetitive tasks such as ticket routing, data entry, or status updates, thus reducing human error and improving efficiency. Below, we explore the considerations for choosing the right automation technology for customer support.
When to Choose AI for Customer Support
- Complex and Unstructured Queries: AI is ideal for handling customer queries that require understanding context, emotions, or open-ended responses.
- Personalized Customer Interactions: AI systems can analyze historical data and tailor responses based on individual customer behavior and preferences.
- Continuous Learning: AI can adapt over time, improving accuracy and performance with each interaction.
When to Choose RPA for Customer Support
- Simple and Repetitive Tasks: RPA is well-suited for automating repetitive, rule-based tasks such as processing requests, updating records, or sending notifications.
- Speed and Accuracy: RPA eliminates manual data entry errors and processes tasks at a much faster pace than human agents.
- Low Maintenance: Once set up, RPA requires minimal intervention for day-to-day operations.
AI is best used when human-like interaction is needed, while RPA excels in automating routine tasks that don’t require judgment or adaptation.
Comparison Table: AI vs RPA for Customer Support
Feature | AI | RPA |
---|---|---|
Task Complexity | Handles complex, unstructured tasks | Handles repetitive, structured tasks |
Learning Ability | Can learn and improve over time | No learning, operates on predefined rules |
Customer Interaction | Human-like conversations and personalized responses | Processes tasks without customer interaction |
Setup and Maintenance | Requires ongoing training and updates | Easy to set up, low maintenance |
When to Choose AI-Driven Chatbots vs. RPA Bots for Automating Tasks
Automation technologies are increasingly being utilized to streamline business operations, and while both AI-driven chatbots and RPA bots offer distinct benefits, their use cases differ significantly. Understanding when to implement each type of bot is essential for maximizing efficiency and achieving the desired outcomes in your processes.
AI-powered chatbots are designed to handle interactions that require understanding of natural language, context, and decision-making. In contrast, RPA bots excel in automating structured, rule-based tasks. Both technologies can complement each other, but the right choice depends on the complexity of the tasks to be automated.
Key Considerations for Choosing Between AI and RPA
AI-Driven Chatbots:
- Designed for customer interaction, responding to queries in real-time.
- Utilize natural language processing (NLP) to understand and process human language.
- Best suited for dynamic, unstructured tasks such as customer support, lead generation, and data gathering.
- Learn and adapt from interactions, making them ideal for evolving tasks over time.
RPA Bots:
- Ideal for repetitive, rule-based tasks with structured data, such as data entry or processing invoices.
- Work with existing applications and systems without requiring changes to infrastructure.
- Perform high-volume tasks with minimal errors and require less human intervention.
- Do not adapt to new situations unless explicitly programmed to do so.
Comparing AI and RPA: A Decision Matrix
Criteria | AI Chatbot | RPA Bot |
---|---|---|
Task Type | Unstructured, dynamic | Structured, repetitive |
Adaptability | Can learn and adapt over time | Fixed process based on rules |
Complexity | High, requires NLP and context understanding | Low, based on predefined rules |
Use Case | Customer support, sales inquiries, personalization | Data entry, report generation, invoice processing |
Choosing the right bot depends on your automation goals. If your processes involve complex decision-making or require ongoing learning, AI chatbots may be the best choice. For straightforward, repetitive tasks, RPA bots will provide a more efficient solution.
The Role of AI in Complex Data Analysis Over RPA in Structured Processes
AI plays a significant role in handling complex data analysis, especially when it involves large volumes of unstructured or semi-structured data. Unlike RPA, which excels in automating repetitive tasks within predefined workflows, AI can interpret and analyze data that doesn't follow strict patterns, enabling deeper insights and decision-making support. AI can process various data formats such as text, audio, and images, leveraging machine learning and natural language processing techniques. This makes AI indispensable for tasks that require cognitive capabilities like pattern recognition, sentiment analysis, or anomaly detection.
In contrast, RPA focuses on streamlining well-defined, rule-based tasks that involve structured data and established workflows. While RPA can significantly improve efficiency in operational processes, its capabilities are limited when the task complexity increases, especially when it comes to non-standardized data. AI, with its ability to adapt and learn from data, provides a more dynamic solution for tasks that involve uncertainty and require advanced decision-making.
Key Differences Between AI and RPA in Data Processing
- Data Flexibility: AI can handle both structured and unstructured data, whereas RPA is best suited for structured, predefined data.
- Learning Capabilities: AI systems improve over time by learning from new data, unlike RPA, which follows pre-programmed rules without deviation.
- Complexity of Tasks: AI is ideal for handling tasks that involve prediction, analysis, and decision-making, while RPA is limited to tasks with a fixed set of rules.
When to Use AI Over RPA
- When dealing with large volumes of unstructured data: AI can process and derive insights from unstructured data sources, like emails or social media.
- For tasks requiring cognitive abilities: AI can perform sentiment analysis, detect anomalies, and predict future outcomes.
- In decision-making processes: AI can assist in complex decision-making by analyzing trends, historical data, and patterns.
AI is essential when the problem at hand requires understanding context, handling ambiguity, or adapting to new situations. RPA, on the other hand, excels in environments with repeatable, predictable processes that follow strict rules.
Comparison Table: AI vs RPA in Data Analysis
Feature | AI | RPA |
---|---|---|
Data Type | Structured and Unstructured | Structured |
Learning Capability | Yes (Machine Learning) | No |
Task Complexity | High Complexity, Decision-making | Low Complexity, Rule-based |
Adaptability | Adapts to New Data | Fixed Process Flow |
Leveraging AI for Dynamic Problem Solving: How It Differs from RPA's Rule-Based Approach
Artificial Intelligence (AI) and Robotic Process Automation (RPA) are both powerful tools in modern automation, but they approach problem-solving differently. While RPA follows predefined rules and performs tasks within a set framework, AI adapts and learns from data, allowing it to handle more complex and dynamic situations. This fundamental difference gives AI a distinct advantage in tasks that require flexibility and context-awareness, unlike RPA's rigid, rule-based structure.
In the following sections, we will explore how AI’s ability to learn and evolve sets it apart from RPA, which relies heavily on a set sequence of actions. Understanding these differences helps organizations choose the right solution depending on the nature of their tasks.
AI vs. RPA: Key Differences in Problem-Solving Approaches
- AI's Learning Capability: AI can learn from historical data and make predictions or decisions based on new, unseen information.
- RPA's Predefined Rules: RPA operates by executing tasks strictly within the boundaries of predefined rules and cannot handle exceptions effectively.
- Adaptability: AI adjusts to changes in the environment or data, whereas RPA requires reprogramming to accommodate any variations in the process.
- Data Processing: AI excels at processing large datasets and identifying patterns, while RPA is limited to task automation without deep data insights.
How AI Solves Problems Dynamically
AI's dynamic problem-solving capability is rooted in machine learning algorithms and neural networks. These systems improve their performance over time as they process more data, adapting to new patterns and information. As a result, AI can make decisions in real-time, optimizing solutions as conditions change.
"AI does not just follow rules; it continuously learns, analyzes, and adjusts its approach, making it suitable for unpredictable and evolving scenarios."
RPA's Rule-Based Approach
RPA, on the other hand, is built for efficiency in performing repetitive tasks. It follows a strict sequence of actions based on predefined rules, which makes it highly efficient for routine processes but limits its effectiveness in more complex or changing environments.
"RPA is highly effective in scenarios where tasks are repetitive and well-defined, but it struggles to adapt to unexpected situations."
Comparison Table: AI vs. RPA in Problem Solving
Feature | AI | RPA |
---|---|---|
Learning Ability | Continuous learning from data | No learning, follows predefined rules |
Adaptability | Adapts to new data and situations | Requires reprogramming for changes |
Task Complexity | Handles complex and dynamic tasks | Performs repetitive and rule-based tasks |
Data Processing | Analyzes large datasets and draws insights | Executes specific tasks without analyzing data |
AI's Practical Impact in Industries vs. RPA's Reach
Artificial Intelligence (AI) and Robotic Process Automation (RPA) have revolutionized various sectors, yet their capabilities and practical applications differ significantly. While RPA automates rule-based tasks, AI enhances decision-making through data processing, pattern recognition, and adaptability. In some industries, AI's ability to learn and adapt has proven more impactful, while RPA thrives in repetitive, high-volume processes that require minimal intervention.
The integration of AI and RPA can often complement each other, enabling businesses to automate complex workflows. AI is more suited for areas requiring cognition and analysis, such as healthcare diagnostics or financial fraud detection, while RPA simplifies manual, time-consuming operations like data entry and invoice processing.
Industries Transformed by AI
- Healthcare: AI models assist in diagnosing diseases, analyzing medical images, and predicting patient outcomes, leading to faster and more accurate diagnoses.
- Finance: AI-driven algorithms detect fraud, manage risks, and optimize trading strategies, providing a competitive edge in investment management.
- Retail: AI enhances personalized customer experiences by analyzing purchasing behaviors and predicting trends, driving customer loyalty and sales growth.
Industries Optimized by RPA
- Banking: RPA automates routine tasks like account reconciliation, loan processing, and customer inquiries, reducing operational costs and improving efficiency.
- Telecommunications: RPA manages data updates, billing operations, and service activation, allowing employees to focus on higher-value tasks.
- Human Resources: RPA streamlines recruitment, employee onboarding, and payroll processing, saving time and reducing human error.
Key Differences in Reach
Aspect | AI | RPA |
---|---|---|
Complexity | Handles cognitive tasks such as prediction, analysis, and decision-making. | Automates repetitive, rule-based tasks with minimal decision-making. |
Adaptability | Can learn from data and improve performance over time. | Works within predefined rules and processes, no learning involved. |
Industries | Most effective in industries requiring advanced data analysis, like healthcare and finance. | Best suited for industries needing high-volume, repetitive tasks, like banking and HR. |
"AI's ability to predict and learn makes it indispensable for industries with complex decision-making, while RPA's efficiency shines in repetitive, structured processes."
Cost Implications of Integrating AI vs RPA in Business Workflows
When considering automation technologies for business operations, the financial aspect is one of the primary factors influencing decision-making. While both Artificial Intelligence (AI) and Robotic Process Automation (RPA) promise significant improvements in efficiency, their cost structures and long-term financial implications differ significantly. Understanding these differences can help businesses make informed choices when selecting the right technology for their workflows.
The investment required for deploying AI solutions is generally higher compared to RPA. AI systems typically require advanced infrastructure, skilled personnel, and ongoing maintenance. On the other hand, RPA tools tend to be less complex and more affordable in the short term, but may not deliver the same level of flexibility or scalability as AI-driven systems.
Key Cost Factors to Consider
- Initial Setup Costs
- AI: Higher due to the need for specialized hardware, software, and expert developers.
- RPA: Lower, as most RPA tools are easier to implement and integrate into existing systems.
- Maintenance & Updates
- AI: Requires continuous updates, especially in machine learning models, which can be costly.
- RPA: Maintenance is less demanding and usually involves simple updates to the scripts.
- Scalability
- AI: More scalable but at a higher incremental cost due to complexity.
- RPA: Can be scaled, but may require significant customization and lead to diminishing returns as tasks become more complex.
Comparison of Financial Implications
Cost Factor | AI | RPA |
---|---|---|
Initial Investment | High | Low |
Ongoing Maintenance | High (due to complexity) | Low (simple updates) |
Scalability | High cost for expansion | Cost-effective for small-scale tasks |
Important: While RPA may be a cost-effective solution for repetitive, rule-based tasks, AI provides greater long-term value for processes that require advanced decision-making capabilities. Businesses should evaluate the scope and complexity of their workflows before deciding on the appropriate technology.