What Is Ai Engine
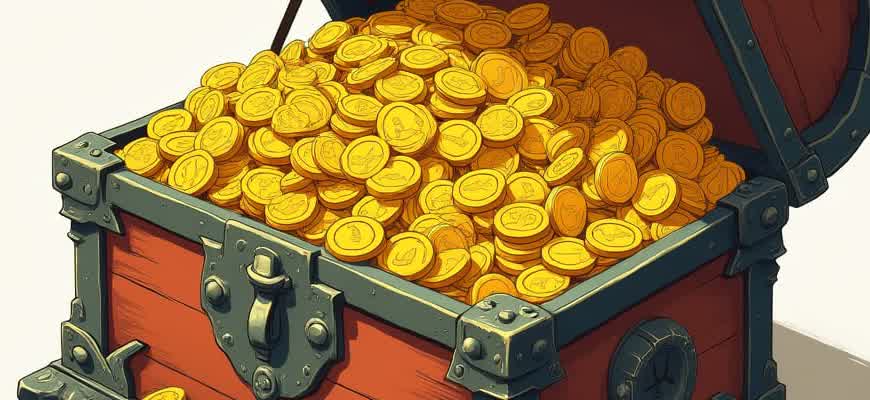
Artificial Intelligence (AI) engines are sophisticated systems designed to process large amounts of data and make decisions or predictions based on that information. These systems leverage algorithms, machine learning models, and vast computing power to simulate human-like reasoning.
AI engines are typically structured in several layers, each serving a distinct purpose in the decision-making process. Some of the core components include:
- Automation: AI engines can automate repetitive tasks, reducing the need for manual labor and lowering operational costs.
- Data Analysis: These tools can analyze vast amounts of data, identifying patterns and insights that might be missed by humans.
- Customer Engagement: AI engines can personalize customer interactions, offering tailored recommendations and improving satisfaction.
- Predictive Capabilities: AI can forecast trends, helping businesses make informed decisions and better prepare for future challenges.
- Algorithms: These mathematical models drive the learning and decision-making processes.
- Data: AI engines rely on large datasets to identify patterns and make accurate predictions.
- Processing Power: Powerful hardware and cloud infrastructure are essential to handle the computing demands of AI tasks.
- Training: AI systems require continuous training to improve their accuracy and performance over time.
- Data Processing: AI engines handle large volumes of structured and unstructured data, streamlining the information collection process.
- Pattern Recognition: AI identifies hidden patterns and correlations in data, making it easier to understand complex datasets.
- Predictive Analytics: By analyzing historical data, AI models can forecast future trends, giving organizations a competitive edge.
- Marketing: AI engines analyze customer behavior, enabling personalized campaigns that drive engagement and conversion.
- Healthcare: AI assists in diagnosing diseases by processing medical data and imaging, improving patient outcomes.
- Finance: AI models detect fraud and assess risks based on transaction patterns and historical financial data.
- Assess Current Processes: Evaluate existing workflows to identify repetitive or time-consuming tasks that AI can improve.
- Data Collection & Cleaning: Ensure high-quality data is available for AI engines to process accurately.
- Choosing the Right AI Tool: Select an AI solution that aligns with your business needs and integrates easily with existing systems.
- Employee Training: Educate staff on how to interact with AI tools, ensuring they understand the value and capabilities of the technology.
- Continuous Monitoring & Optimization: Regularly assess AI performance and make adjustments to improve outcomes.
- Resistance to Change: Employees may be hesitant to adopt new technologies, fearing job displacement or the complexity of the systems.
- Data Compatibility: Integrating AI systems often requires data to be in a specific format or structure, which can be a challenge for businesses with inconsistent data practices.
- Cost and Resources: Implementing AI solutions can be resource-intensive, requiring investment in technology, infrastructure, and skilled personnel.
- Industry-specific features: Some engines are tailored to specific sectors, like healthcare, retail, or finance. Make sure the AI engine supports the nuances of your industry.
- Scalability: Can the engine scale as your business grows? Look for platforms that can handle increased data loads and more complex tasks as your needs evolve.
- Integration with existing tools: Consider how well the AI engine integrates with your current software stack, including CRM, ERP, or other business management systems.
- Cost and licensing: Assess the total cost of ownership, including licensing, deployment, and maintenance fees.
- Identify your core requirements: Define your business needs in terms of the AI capabilities required (e.g., data analysis, automation, personalization).
- Evaluate potential engines: Research and compare AI engines that align with your needs. Look for case studies, reviews, and expert opinions.
- Run a pilot project: Test the engine with a small-scale project to assess its performance, integration, and overall effectiveness.
- Consult with experts: Seek advice from AI consultants or industry peers to validate your choice.
- Personalized Recommendations: AI engines analyze browsing behavior and past interactions to suggest products or services aligned with individual preferences.
- Instant Customer Support: Chatbots and virtual assistants powered by AI can respond to inquiries and resolve issues 24/7 without human intervention.
- Predictive Analytics: By processing large datasets, AI engines predict customer needs and proactively address potential issues before they occur.
- Increased Customer Loyalty: Personalized experiences foster trust, encouraging repeat business.
- Improved Operational Efficiency: AI systems reduce the need for manual intervention, leading to faster resolutions and lower operational costs.
- Higher Conversion Rates: By offering relevant suggestions at the right moment, AI engines drive higher conversion rates in e-commerce and sales.
- Data Collection and Preparation: The first step involves gathering relevant and high-quality data. This data must be cleaned, normalized, and preprocessed to eliminate inconsistencies and ensure it is ready for training.
- Model Selection: Choosing the right type of model is critical. Depending on the problem, you may choose from various algorithms like decision trees, neural networks, or support vector machines.
- Training the Model: This step involves feeding the prepared data into the selected model and allowing it to learn patterns. The model adjusts its internal parameters through iterative optimization techniques.
- Model Evaluation: After training, it's essential to evaluate the model's performance using a test set. Key metrics such as accuracy, precision, recall, and F1 score are used to assess how well the model predicts new data.
- Overfitting: When a model learns the training data too well, it may fail to generalize to new data, resulting in poor performance on unseen cases.
- Underfitting: If the model is too simple, it may not capture the underlying patterns in the data, leading to inaccurate predictions.
- Bias in Data: Incomplete or biased data can skew the model's predictions and lead to unfair or inaccurate results.
- Improved Decision-Making: AI systems analyze large datasets and provide actionable insights that assist in making more informed and faster decisions.
- Cost Efficiency: By automating repetitive tasks, businesses can significantly reduce labor costs and focus resources on higher-value activities.
- Consistency and Accuracy: Unlike humans, AI engines are less prone to errors, ensuring a higher level of precision in task execution.
- Manufacturing: AI-powered robots can optimize assembly lines, reduce waste, and improve quality control processes.
- Customer Support: AI chatbots handle a wide range of customer inquiries, providing quick responses and freeing up human agents for more complex cases.
- Logistics: AI engines can predict demand, optimize routes, and manage inventory, ensuring timely deliveries and reducing operational costs.
- Initial Development and Integration: Building or customizing an AI engine to meet specific business needs requires significant development time and resources.
- Infrastructure Requirements: AI systems often demand powerful hardware and cloud services, adding to the cost of implementation.
- Training and Maintenance: Continuous learning and system updates are needed to ensure the AI remains relevant and effective.
- Increased Efficiency: AI can automate repetitive tasks, freeing up human resources for more complex work.
- Data-Driven Decisions: AI helps in analyzing large datasets quickly, leading to faster and more accurate decision-making.
- Scalability: AI solutions can scale easily as business needs grow, providing long-term value.
- Advanced Natural Language Processing (NLP): AI engines will better understand and process human language, enhancing customer interactions and providing more efficient automated services.
- AI-Driven Automation: As AI engines become more capable, automation across various sectors will improve, driving down costs and increasing productivity in industries like manufacturing and logistics.
- Explainable AI (XAI): With businesses relying more on AI for decision-making, there will be a greater emphasis on developing AI systems that can explain their reasoning in an understandable way, ensuring transparency and trust.
- Personalized Marketing: AI engines can analyze consumer behavior and create customized marketing strategies, increasing engagement and conversion rates.
- Predictive Analytics: AI will enable businesses to anticipate future trends, forecast demand, and optimize inventory management through data-driven insights.
- Healthcare Innovations: AI will support medical research, patient diagnosis, and personalized treatments, enhancing healthcare outcomes and reducing costs.
What Is an AI Engine? A Practical Guide for Businesses
An AI engine is a set of software tools and algorithms designed to simulate human intelligence processes. These engines help businesses to analyze data, make predictions, automate tasks, and improve decision-making. They often rely on machine learning, deep learning, and natural language processing to perform specific functions. As technology advances, AI engines are becoming increasingly capable of handling complex business problems across various industries.
For businesses, implementing an AI engine can significantly streamline operations, boost efficiency, and enhance customer experiences. Whether it's automating customer support, analyzing consumer behavior, or optimizing supply chains, AI engines provide the backbone for intelligent business solutions. Understanding how AI engines work is crucial for organizations that want to stay competitive in today's rapidly evolving market.
How AI Engines Benefit Businesses
Key Components of an AI Engine
Important: Businesses must have a clear strategy when adopting an AI engine. It is essential to define objectives, prepare quality data, and regularly evaluate system performance.
Choosing the Right AI Engine for Your Business
Criteria | Importance |
---|---|
Customization | Ensures the AI engine can be tailored to specific business needs. |
Scalability | AI solutions should scale as the business grows, handling increasing data and demand. |
Cost | Consider the total cost of implementation, including software, hardware, and maintenance. |
Integration | Ensure the AI engine can integrate seamlessly with existing systems and workflows. |
How AI Engines Drive Data-Driven Decision Making
AI engines have become essential tools for businesses and organizations aiming to leverage vast amounts of data to make informed decisions. These systems are built to process and analyze massive datasets, extracting patterns, insights, and predictions that would be nearly impossible for humans to identify manually. By automating the process of data analysis, AI engines help improve decision-making efficiency, accuracy, and scalability.
AI-driven decision-making empowers companies to not only understand historical trends but also predict future outcomes, which allows for more strategic planning and resource allocation. The ability to swiftly process and interpret data in real time enables businesses to make responsive, data-backed choices that can optimize operations and drive growth.
Key Roles of AI in Data-Driven Decisions
Examples of AI-Powered Decision Making
Impact on Decision Quality
AI-enhanced decision-making offers superior accuracy and agility, reducing human error and biases while offering actionable insights that are more reliable and timely.
Comparison of Traditional vs. AI-Driven Decision Making
Aspect | Traditional Decision Making | AI-Driven Decision Making |
---|---|---|
Speed | Slow, often reliant on human analysis | Fast, powered by real-time data processing |
Accuracy | Susceptible to human error and biases | More accurate, based on data and algorithms |
Scalability | Limited by human capacity and time | Scalable to handle vast datasets |
Integrating AI Engines into Existing Business Workflows
Integrating artificial intelligence (AI) into established business processes requires a thoughtful approach to ensure compatibility and maximize efficiency. AI can significantly enhance workflows by automating repetitive tasks, providing advanced analytics, and improving decision-making. However, integrating AI tools into an organization’s existing systems demands careful planning and a structured implementation strategy. It's essential to assess the specific needs of the business and how AI can seamlessly blend with the current infrastructure.
Successful AI integration involves aligning AI capabilities with business objectives, ensuring minimal disruption, and training employees to work with the new technologies. The process typically involves several key stages, including system evaluation, data preparation, algorithm selection, and continuous optimization. By strategically incorporating AI into workflows, businesses can unlock new opportunities for growth and operational efficiency.
Key Steps for Successful AI Integration
Challenges of AI Integration
Example: AI in Customer Support
Traditional Workflow | AI-Enhanced Workflow |
---|---|
Customer service representatives handle all inquiries manually. | AI chatbots triage inquiries, escalating complex issues to human agents. |
Customer queries are logged and responded to through emails or calls. | AI tools track customer sentiment and suggest personalized responses in real-time. |
Data is stored in siloed systems, making it difficult to get a unified view of customer interactions. | AI integrates data from multiple channels, offering a comprehensive view of customer behavior and preferences. |
"AI engines can transform how businesses operate, but careful planning and strategic implementation are key to achieving successful integration and long-term benefits."
Choosing the Right AI Engine for Your Industry Needs
When selecting an AI engine, it is crucial to consider the specific requirements of your industry. Different sectors have unique demands, whether it be for automation, predictive analytics, natural language processing, or image recognition. Understanding the technical capabilities, scalability, and ease of integration of the AI platform will ensure that it meets your operational goals effectively.
In addition to functionality, evaluating the support and community around an AI engine can significantly impact your implementation. A well-supported engine can streamline troubleshooting, while a large user base can provide valuable insights into best practices. Below are key factors to consider when selecting the ideal AI engine for your industry.
Key Considerations for Selecting an AI Engine
Steps for Choosing the Right AI Engine
"Choosing the right AI engine can transform your business operations. The right fit not only improves efficiency but also provides a competitive edge in your industry."
AI Engine Comparison Table
AI Engine | Key Features | Best For |
---|---|---|
TensorFlow | Open-source, flexible, supports deep learning | Data science, complex model training |
IBM Watson | Natural language processing, AI-powered analytics | Customer service, healthcare analytics |
Google Cloud AI | Machine learning, vision, speech recognition | Large-scale data processing, cloud integration |
How AI Engines Optimize Customer Experience in Real-Time
AI engines play a pivotal role in enhancing customer experience by delivering real-time, personalized interactions. These systems analyze vast amounts of data from customer behavior, preferences, and interactions to dynamically adjust and provide tailored experiences. By leveraging advanced algorithms and machine learning, AI engines ensure that businesses can meet the needs of customers instantly, improving satisfaction and engagement.
In real-time, AI engines can anticipate customer actions and provide relevant recommendations, streamline processes, and solve problems proactively. This capability allows companies to address customer issues swiftly, reduce wait times, and create a seamless, personalized journey for every user.
Key Mechanisms AI Engines Use for Optimization
Example of AI-Driven Real-Time Optimization
Real-time optimization allows companies to adjust their approach based on immediate customer data, ensuring a high level of satisfaction and efficiency during every interaction.
Feature | Benefit |
---|---|
Behavior Analysis | Identifies patterns to deliver personalized content in real-time. |
Real-Time Interaction | Improves engagement by responding instantly to customer queries. |
Automation | Reduces human error and accelerates customer service processes. |
Impact on Business Performance
AI Engine Training: Key Steps to Ensure Accurate Predictions
Training an AI engine is a crucial process for achieving reliable and precise predictions. It involves a series of well-defined steps that ensure the model can generalize well to new, unseen data. Understanding these steps is essential for engineers and data scientists who aim to build robust AI systems. The following sections break down these steps in detail.
To guarantee the accuracy of predictions made by AI engines, it's necessary to follow a structured training methodology. Each phase contributes to the overall performance of the model and its ability to adapt to real-world situations. Below are key stages to consider when training an AI engine.
Key Steps in AI Engine Training
"The quality of training data directly impacts the reliability of the predictions generated by the AI engine."
Common Challenges in AI Training
Training Process Overview
Step | Action | Goal |
---|---|---|
Data Preparation | Collect and clean data | Ensure high-quality input data |
Model Selection | Choose appropriate model type | Match model to problem |
Model Training | Train using data and optimize | Achieve accurate parameter values |
Evaluation | Test model performance | Verify prediction accuracy |
Understanding the Impact of AI Engines on Automation Processes
AI engines play a crucial role in enhancing automation workflows by providing advanced capabilities for data analysis, decision-making, and task execution. These systems are designed to mimic human cognitive functions, which enables them to process complex information and automate tasks that were previously manual or labor-intensive. Their integration into business processes has revolutionized industries, offering increased efficiency and reduced operational costs.
Automation powered by AI engines is not just about speed; it’s about precision, adaptability, and scalability. AI systems continuously learn from data, improving their performance over time. This ability to evolve makes them especially valuable in dynamic environments where conditions change rapidly, such as in manufacturing, logistics, and customer service.
Key Benefits of AI Engines in Automation
Examples of Automation Powered by AI Engines
"AI engines empower automation processes to be more adaptive, precise, and scalable, driving businesses towards higher productivity and innovation."
Challenges in Integrating AI Engines
Challenge | Impact |
---|---|
Data Quality | AI engines rely on accurate, high-quality data to function effectively. Poor data can lead to inaccurate results and decisions. |
Cost of Implementation | The initial investment in AI infrastructure can be substantial, which may be a barrier for some organizations. |
Workforce Displacement | Automation may lead to job displacement in some sectors, requiring reskilling and upskilling of the workforce. |
Evaluating the Costs and Benefits of Implementing an AI Engine
When considering the implementation of an AI engine in a business or operational environment, it’s important to assess both the potential costs and benefits that come with such an investment. The decision should be based not only on short-term financial implications but also on long-term strategic advantages. AI can provide automation, enhance decision-making, and streamline operations, but the initial and ongoing costs can be substantial. Understanding these factors is crucial for any organization looking to integrate AI into their processes.
From a financial perspective, implementing an AI system involves various expenses, such as development, infrastructure, and training costs. However, the return on investment (ROI) can manifest in increased efficiency, improved customer experiences, and a competitive edge in the market. The balance between these costs and benefits must be carefully considered to determine the overall impact on the business.
Key Costs of Implementing an AI Engine
Benefits of Integrating an AI Engine
Summary Table
Cost | Benefit |
---|---|
Initial investment in infrastructure | Improved productivity and reduced operational costs |
Continuous maintenance and updates | Enhanced data insights and smarter decision-making |
Training for employees | Increased scalability and flexibility |
While the upfront costs of AI implementation may seem high, the long-term benefits in terms of efficiency and data analysis can significantly outweigh these initial expenditures.
Future Trends in AI Engine Development and Their Business Applications
As artificial intelligence continues to evolve, its engines are becoming increasingly sophisticated. This development will significantly impact how businesses operate, enhancing efficiency, personalization, and decision-making. AI-driven systems are expected to become more intuitive, with the ability to understand complex human interactions and adapt to diverse business needs. In the near future, we can expect AI engines to drive major transformations in various sectors, creating opportunities for companies to gain a competitive edge.
Key advancements in AI engine development will focus on improving cognitive capabilities, increasing scalability, and reducing operational costs. These improvements are set to revolutionize industries ranging from healthcare to finance, enabling businesses to streamline processes, personalize customer experiences, and automate routine tasks. Below are some key trends to look out for in the development of AI engines:
Key Trends in AI Engine Development
Business Applications of AI Engines
"As AI technology matures, businesses that adopt advanced AI engines will be better equipped to handle increasing complexity and achieve long-term success in the digital economy."
AI Engines in the Workplace: A Look at Automation
AI engines are not only transforming customer-facing applications but also revolutionizing internal business processes. Automation powered by AI will improve back-office operations, reduce human error, and optimize workflows. Companies that integrate AI into their supply chain, human resources, and financial operations will experience significant gains in efficiency and cost reduction.
AI Engine Application | Industry Impact |
---|---|
Customer Service Automation | Improved customer satisfaction, reduced response times |
Predictive Maintenance | Reduced downtime and maintenance costs in manufacturing |
Fraud Detection | Enhanced security in finance and e-commerce sectors |