How Much Does It Cost to Build an Ai
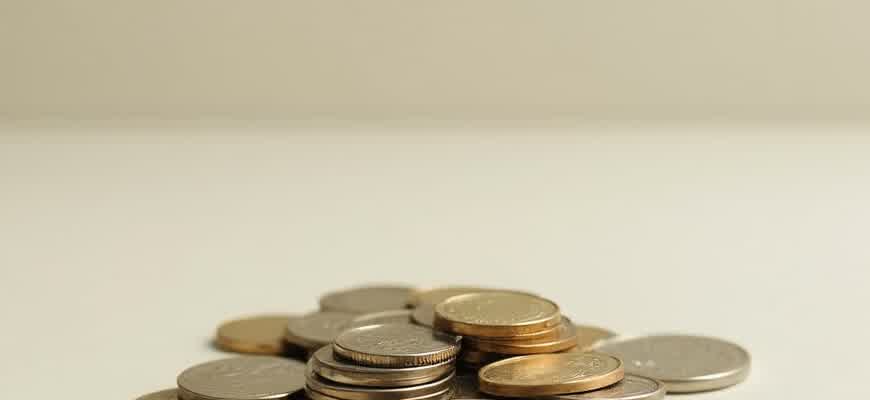
Building an AI system involves several components, each with its own financial implications. The overall expense can vary significantly depending on the scale, complexity, and type of AI project you're working on. Below are the key factors that contribute to the cost of creating an AI solution.
- Data Acquisition: Collecting and preparing high-quality data is often one of the most expensive and time-consuming parts of AI development.
- Software Development: Custom algorithms and models need to be developed or adapted to fit specific business requirements.
- Hardware Requirements: Powerful computing resources, including GPUs and specialized hardware, are necessary for training AI models.
- Expert Personnel: AI engineers, data scientists, and domain experts are essential to building and fine-tuning AI systems.
Let's take a closer look at some of these costs:
Important Note: AI development is not a one-time investment. Continuous maintenance, updates, and improvements are needed over time.
Cost Breakdown by Project Type
Project Type | Estimated Cost |
---|---|
AI for Data Analysis | $50,000 - $100,000 |
AI for Computer Vision | $100,000 - $500,000 |
AI for Natural Language Processing | $200,000 - $1,000,000 |
AI for Autonomous Systems | $500,000 - $5,000,000 |
Understanding the Expenses of AI Development
Building artificial intelligence (AI) involves various stages and resources, which significantly impact the overall cost. The expenses can vary based on the scope of the project, the complexity of the AI model, and the technology stack used. In this context, AI development typically requires investment in infrastructure, data acquisition, algorithm design, and specialized talent. Companies need to assess both direct and indirect costs when budgeting for AI initiatives.
The costs associated with building AI can range from a few thousand dollars for simple AI tools to millions of dollars for more complex systems. Below is an overview of the key cost drivers in AI development.
Key Cost Factors in AI Development
- Infrastructure Costs: High-performance computing resources, cloud services, and data storage systems.
- Data Acquisition: Gathering and preparing high-quality data is crucial and often requires significant investment.
- Talent and Expertise: AI specialists such as data scientists, machine learning engineers, and domain experts are in high demand, making their salaries a major cost factor.
- Algorithm Development: Developing and fine-tuning algorithms for specific applications is a time-consuming and resource-heavy process.
AI Project Budget Breakdown
Category | Estimated Cost |
---|---|
Infrastructure (Cloud Computing, Servers) | $10,000 - $200,000+ |
Data Acquisition and Preparation | $5,000 - $50,000+ |
AI Talent (Salaries of Engineers, Scientists) | $100,000 - $500,000+ |
Algorithm Development | $20,000 - $100,000+ |
Note: The cost of AI development can vary greatly depending on the complexity and scale of the project, and these are general estimates for small to medium-sized projects.
Initial Expenses for AI Development: Key Considerations
When embarking on an AI project, the upfront investment can vary significantly based on the project's scope, technology stack, and team expertise. Understanding the core components involved in the initial development phase is essential for estimating costs. These costs will be shaped by several factors, including infrastructure, talent, and the specific tools required for machine learning and AI models.
The initial expenses can be broken down into several key categories that contribute to the overall budget. This will give you a clearer understanding of where resources are allocated during the early stages of AI development.
Key Components of Initial AI Development Costs
- Personnel and Expertise: Salaries for AI specialists such as data scientists, machine learning engineers, and researchers. Their experience and skillset greatly impact the cost.
- Technology Infrastructure: Computing resources such as GPUs, cloud computing services, and storage solutions are crucial for training AI models effectively.
- Data Acquisition and Preparation: The cost of sourcing, cleaning, and preparing data for training AI models is often a significant part of the initial phase.
- Software and Tools: Licensing costs for specialized AI software, frameworks, and libraries can add up quickly.
Estimated Breakdown of Initial Costs
Expense Category | Estimated Cost |
---|---|
Personnel (AI Specialists) | $80,000 - $150,000 per year |
Cloud Computing (Storage, GPUs) | $10,000 - $50,000 |
Data Acquisition and Preparation | $5,000 - $30,000 |
Software Licensing | $2,000 - $15,000 |
Investing in the right talent and infrastructure during the initial stages can significantly influence the success of your AI project in the long run.
Factors That Affect the Cost of Developing AI Projects
When considering the development of an artificial intelligence system, multiple elements contribute to its overall cost. These factors include the complexity of the project, the required infrastructure, and the expertise of the development team. By evaluating each of these components, businesses can get a more precise estimate of the financial investment needed. Understanding these influences is crucial for both small startups and large corporations planning to implement AI solutions.
AI projects vary significantly in price due to the specific goals of the system, the resources required, and the scope of implementation. These projects typically fall into categories based on their scope, which directly influences costs. Below are key aspects that businesses should consider when budgeting for AI development.
Key Factors Affecting AI Development Costs
- Project Scope and Complexity: The scale and intricacy of the AI system greatly impact the cost. A complex system requiring advanced machine learning models or natural language processing will be more expensive to develop than a simpler rule-based AI.
- Data Requirements: Data is the backbone of AI, and acquiring quality datasets can be both time-consuming and costly. The need for clean, large-scale, and domain-specific data increases project expenses.
- Development Team Expertise: Highly skilled data scientists, engineers, and AI specialists command premium salaries. The more experienced the team, the higher the costs.
- Hardware and Infrastructure: The computational power required to train AI models, including GPUs and cloud infrastructure, can contribute to substantial costs, especially for large-scale projects.
- Project Timeline: Shorter development timelines can lead to higher costs, as more resources are needed to meet tight deadlines.
Detailed Breakdown of Cost Elements
Factor | Cost Impact |
---|---|
Scope and Complexity | Higher complexity increases costs due to more advanced models and longer development cycles. |
Data Acquisition | Data gathering, cleaning, and preprocessing require significant investment in time and resources. |
Team Expertise | Specialized AI talent costs more, raising the overall project budget. |
Hardware and Infrastructure | High-performance computing resources like GPUs and cloud services add to the budget. |
Important: AI projects with a more narrow, well-defined purpose tend to be less expensive. In contrast, systems requiring deep learning or reinforcement learning models are more costly due to the specialized knowledge and computational power needed.
Comparing AI Development Costs: In-House vs. Outsourcing
When deciding whether to build an AI solution internally or outsource it to a third-party provider, the costs can vary greatly depending on the approach you take. The decision typically revolves around the long-term investment, skill requirements, and the complexity of the AI project. In-house development offers full control over the project, while outsourcing can save time and reduce overhead costs by leveraging external expertise.
Both options have distinct advantages and challenges, and understanding the financial implications is crucial for businesses looking to implement AI systems effectively. Below, we compare the costs associated with in-house development and outsourcing through key factors.
Cost Breakdown: In-House vs. Outsourcing
- Team Setup: In-house development requires a dedicated team of developers, data scientists, and AI specialists. These roles can be costly due to high salaries and the need for specialized skills.
- Infrastructure: Building AI in-house means investing in the right infrastructure, including hardware, software, and cloud services. Outsourcing usually means less upfront cost, as providers already have the necessary infrastructure in place.
- Time to Market: In-house teams may face longer development times, especially if the necessary expertise is not readily available. Outsourcing can speed up the process due to the provider’s experience and resources.
In-house teams may offer more control and customization, but outsourcing often provides quicker access to specialized knowledge, allowing for faster development cycles.
Cost Comparison Table
Cost Factor | In-House Development | Outsourcing |
---|---|---|
Initial Setup | High (team salaries, infrastructure) | Moderate (contractor fees, minimal infrastructure investment) |
Operational Costs | Continuous (salaries, tools, training) | Variable (project-based, maintenance fees) |
Speed of Development | Slower (dependent on internal resources) | Faster (external expertise and resources) |
Ultimately, the choice between in-house development and outsourcing depends on the specific needs of the business and the complexity of the AI solution. Outsourcing can be a cost-effective solution for short-term projects, while in-house teams may be better suited for long-term, highly customized AI systems.
The Role of Data Collection and Preprocessing in AI Cost
The process of collecting and preparing data is crucial when developing artificial intelligence models. The quality and quantity of the data used significantly influence the accuracy of the model, as well as the resources required for its creation. The cost of gathering, cleaning, and organizing data can quickly add up, depending on the scale and complexity of the project. This stage represents a significant portion of the overall AI development cost due to the time, personnel, and computational power required.
Data preprocessing includes tasks such as data cleaning, normalization, transformation, and feature extraction. These activities are necessary to ensure that the data is usable and that the AI model can learn effectively. In many cases, this process requires a combination of manual effort and automated tools. The larger and more diverse the dataset, the more time and money it takes to prepare the data for training an AI model.
Key Costs in Data Collection and Preprocessing
- Data Acquisition: Costs related to purchasing datasets or collecting raw data through surveys, sensors, web scraping, or public APIs.
- Data Cleaning: Identifying and removing incomplete, incorrect, or irrelevant data points to ensure data quality.
- Data Transformation: Converting raw data into a format suitable for machine learning algorithms, including normalization, encoding, or feature scaling.
- Data Labeling: Manually tagging data for supervised learning, which is often time-consuming and requires human effort.
Stages of Data Preparation
- Collection: Gathering data from various sources, ensuring diversity and relevance to the problem at hand.
- Cleaning: Removing errors, duplicates, and inconsistencies in the data.
- Transformation: Converting data into a structured and standardized form.
- Labeling: Annotating data for supervised learning tasks.
Data preprocessing is an essential step to building high-quality AI models. Poorly prepared data can lead to inaccurate models, making this process not only time-consuming but also costly.
Budget Breakdown for Data Preparation
Task | Cost Estimate |
---|---|
Data Acquisition | $1,000 - $50,000+ |
Data Cleaning | $500 - $10,000+ |
Data Transformation | $500 - $5,000+ |
Data Labeling | $2,000 - $50,000+ |
How AI Algorithms Influence the Total Development Costs
When developing artificial intelligence (AI) solutions, the choice of algorithms significantly affects the overall budget. The complexity, performance requirements, and the need for specialized expertise all contribute to increased costs. AI models, ranging from simple regression to deep learning networks, each have their own set of resource demands, which can substantially drive up the cost of development.
Moreover, the type of algorithm used often determines the time and financial investment needed for training, optimization, and deployment. Some AI models require large-scale data, powerful hardware, and extended processing time, all of which add to the total development expenses.
Key Factors Affected by AI Algorithms
- Data Collection and Preprocessing: More complex algorithms often require larger and more diverse datasets. The cost of acquiring and cleaning such data can be significant.
- Computational Power: Advanced AI techniques, especially deep learning, demand high-performance hardware (e.g., GPUs, TPUs), which increases infrastructure costs.
- Development Time: Some algorithms, particularly those involving deep neural networks, require longer periods for training and fine-tuning, driving up labor costs.
- Expertise: Specialized knowledge in fields like machine learning or reinforcement learning is required to implement and optimize certain algorithms, which may necessitate hiring high-end experts or consultants.
Algorithm Types and Their Budget Impact
Algorithm Type | Cost Impact | Resource Demands |
---|---|---|
Linear Regression | Low | Minimal data and computational resources |
Decision Trees | Moderate | Moderate data and computational resources |
Deep Learning (Neural Networks) | High | Large datasets, high computational power |
Reinforcement Learning | Very High | Extensive computational resources, complex model tuning |
Note: The more complex the AI algorithm, the more resources and time it typically requires for training, validation, and optimization. This directly correlates with the overall project cost.
Unseen Costs When Developing AI: Key Considerations
Building artificial intelligence (AI) systems often comes with more expenses than initially anticipated. While the main costs, such as hardware, software, and talent acquisition, are commonly discussed, there are several hidden expenses that developers and businesses may overlook. These hidden costs can quickly add up, impacting the overall budget and timeline of AI projects.
Understanding these hidden expenses is crucial for managing resources effectively. Below are several factors that can lead to unforeseen costs during AI development.
Key Hidden Expenses
- Data Acquisition and Cleaning: Sourcing high-quality, relevant datasets can be expensive. Data often needs to be cleaned and pre-processed, which can require significant resources and time.
- Ongoing Maintenance and Updates: AI systems need continuous monitoring and fine-tuning to maintain their accuracy and relevance. This may require frequent updates, adjustments to algorithms, and troubleshooting.
- Computational Power: Training AI models, especially deep learning models, demands substantial computational power, which translates into high cloud computing or hardware infrastructure costs.
- Compliance and Legal Fees: AI development, particularly with data privacy regulations, may incur legal costs for ensuring compliance with GDPR or other regulatory frameworks.
Examples of Hidden Costs
Expense | Potential Cost Impact |
---|---|
Data Acquisition | Cost of purchasing proprietary datasets or collecting data from various sources |
Model Tuning | Additional time and resources to optimize models for better performance |
Hardware Infrastructure | Upfront investment or ongoing cloud costs for GPU and server usage |
Many businesses overlook the costs associated with ensuring data quality and the technical debt that builds up during the AI lifecycle. These hidden costs can impact the final output, leading to increased expenses over time.
Conclusion
Hidden costs in AI development can be challenging to estimate, but understanding and planning for these factors is essential. While the upfront costs of AI projects can be considerable, the ongoing expenses related to data, infrastructure, and legal requirements can significantly affect the overall budget.
Ongoing Maintenance and Updates: Costs Beyond the Initial Build
When it comes to developing AI systems, many organizations focus on the initial creation and overlook the long-term costs associated with maintaining and updating the system. Building an AI solution requires a significant investment, but its true cost is not limited to the development phase. Continuous updates, performance monitoring, and adapting the model to new data all contribute to the ongoing expenses of maintaining a robust AI system.
The ongoing costs can be categorized into several areas. These costs depend on factors such as the complexity of the AI, the rate at which updates are needed, and the infrastructure required to support the system. Here are the key aspects to consider:
Key Areas of Ongoing AI Maintenance Costs
- Model Retraining and Fine-Tuning: AI models need regular updates to stay relevant. This includes retraining with new data to ensure accuracy and performance. The frequency of this depends on the specific AI application.
- Cloud Infrastructure and Data Storage: AI systems often rely on cloud-based platforms for processing and storing large amounts of data. These services have recurring costs for storage and computational power.
- Monitoring and Performance Optimization: Continuous monitoring is required to ensure that the AI performs optimally. This includes identifying and resolving any performance bottlenecks or issues as they arise.
Detailed Breakdown of Maintenance Costs
Cost Factor | Estimated Cost |
---|---|
Model Retraining | $10,000 - $100,000 annually |
Cloud Services (Storage & Compute) | $5,000 - $50,000 monthly |
Monitoring and Support | $2,000 - $20,000 monthly |
Regular updates to AI models and infrastructure are necessary to keep them efficient and secure, but these can significantly increase the operational costs beyond the initial build.
Challenges in Managing Ongoing AI Costs
- Scaling and Adaptation: As AI systems scale, the costs of maintaining them grow, especially when new features or capabilities are added.
- Data Management: Proper data management is essential to keep the system working effectively. This includes regular data cleaning, labeling, and validation.
- Security Updates: AI systems must be updated with the latest security patches to prevent vulnerabilities and data breaches.
Evaluating the Price of AI Solutions: Which Ones Justify the Cost?
When building an AI system, the costs associated with the tools and platforms you choose can vary significantly. While some solutions offer a broad set of capabilities, others are more niche-focused, offering specialized features. It's crucial to assess the specific needs of your project before investing in any platform or tool. This helps determine whether the tool will deliver a return on investment (ROI) based on performance, scalability, and ease of integration into your workflow.
AI tools come with different pricing models, ranging from pay-per-use to subscription-based options. When evaluating their worth, businesses should weigh the potential benefits, such as advanced machine learning models, ease of use, and customer support. Below, we'll look at some popular AI tools and platforms, alongside factors that can impact their value for money.
Cost-Effective AI Platforms and Their Key Features
- Google Cloud AI: Offers a broad array of machine learning tools, including pre-trained models and AutoML. Best for scalability and integration with Google Cloud infrastructure.
- AWS AI Services: Provides a comprehensive set of AI tools, such as AWS SageMaker for building models, with a pay-as-you-go pricing structure.
- Microsoft Azure AI: Known for its robust support for enterprise solutions, offering tools for AI, machine learning, and data processing at competitive rates.
"Investing in a platform with flexible pricing allows businesses to scale their AI solutions as they grow, avoiding unnecessary upfront costs."
AI Tools Comparison: Price vs. Features
Tool/Platform | Key Features | Pricing Model | Best For |
---|---|---|---|
Google Cloud AI | Pre-trained models, AutoML, scalable infrastructure | Pay-per-use | Scalability and integration with Google services |
AWS AI Services | SageMaker, Natural Language Processing, Computer Vision | Pay-per-use, subscription | Flexible pricing for various AI projects |
Microsoft Azure AI | Enterprise-level AI, robust machine learning tools | Subscription, pay-as-you-go | Large enterprises needing reliable, secure AI solutions |
Key Considerations When Deciding on AI Investment
- Project Scope: Determine if the platform's features align with your project requirements.
- Scalability: Look for platforms that grow with your needs without requiring massive upfront investment.
- Support and Documentation: Comprehensive support and a robust knowledge base can significantly reduce long-term costs.