How to Use Ai and Ml in Business
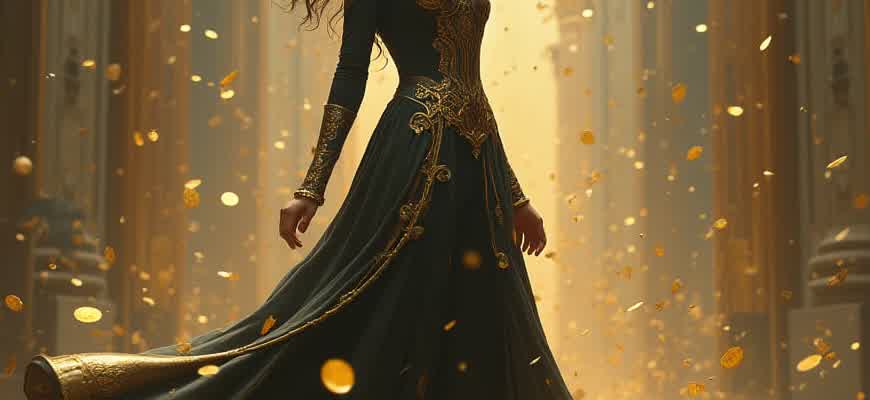
Artificial Intelligence (AI) and Machine Learning (ML) have emerged as transformative technologies that businesses can integrate to optimize operations, improve customer experiences, and drive innovation. These technologies enable companies to automate processes, analyze vast amounts of data, and make data-driven decisions. Below are key ways businesses can incorporate AI and ML into their operations:
- Data Analytics and Predictive Insights - Machine learning models can analyze customer behavior and predict future trends, allowing businesses to make informed decisions based on data-driven insights.
- Automation of Repetitive Tasks - AI-driven tools can automate routine administrative tasks, reducing the workload on human employees and improving operational efficiency.
- Enhanced Customer Service - Chatbots and virtual assistants powered by AI can provide 24/7 customer support, addressing queries and improving customer satisfaction.
To implement these technologies successfully, businesses should take a structured approach:
- Identify Business Needs - Evaluate areas where AI and ML can add the most value, such as customer insights, process automation, or product development.
- Invest in Data Infrastructure - Build a robust data ecosystem that enables seamless data collection, processing, and analysis.
- Adopt Scalable AI Solutions - Choose AI and ML tools that can scale with the growth of the business and adapt to evolving needs.
Key Consideration: Success in AI and ML adoption requires strong collaboration between data scientists, business analysts, and decision-makers to ensure that the solutions align with business goals.
Table: Examples of AI and ML Applications in Business
Application | Description |
---|---|
Customer Personalization | AI algorithms analyze user data to deliver personalized recommendations and targeted marketing campaigns. |
Fraud Detection | Machine learning models identify fraudulent transactions by analyzing patterns and anomalies in data. |
Supply Chain Optimization | AI tools optimize inventory management, demand forecasting, and logistics planning for improved efficiency. |
How to Leverage Artificial Intelligence and Machine Learning in Business
Artificial Intelligence (AI) and Machine Learning (ML) are transforming the way businesses operate by enhancing decision-making, improving customer experiences, and automating complex tasks. By adopting AI and ML, companies can gain a competitive advantage through data-driven insights and more efficient processes.
Implementing AI and ML technologies requires a strategic approach, starting with identifying business needs, selecting the right tools, and then integrating them into existing workflows. Below are the key steps to effectively use these technologies in business.
Key Steps to Implement AI and ML in Business
- Define the Problem: Before implementing AI/ML, clearly identify the business challenge or opportunity. Understanding the exact problem helps in selecting the right algorithm and approach.
- Choose the Right Technology: Select AI or ML tools that best suit your needs. Popular options include supervised learning for predictive tasks or unsupervised learning for clustering and pattern recognition.
- Data Collection and Preparation: AI and ML models require high-quality data. Ensure data is cleaned, organized, and relevant to the problem at hand.
- Model Training and Testing: Train the model using historical data, then test it to evaluate its performance and make necessary adjustments.
- Deploy and Monitor: Once tested, deploy the model into real-world business processes and continuously monitor its performance for further optimization.
“AI and ML are not just tools; they are business accelerators that enhance productivity and unlock new opportunities.”
Examples of AI and ML Applications in Business
- Customer Service: AI-powered chatbots and virtual assistants can handle customer inquiries, improving efficiency and reducing human workload.
- Sales and Marketing: ML algorithms can analyze customer data and predict purchasing behavior, allowing businesses to target marketing efforts more effectively.
- Supply Chain Optimization: AI can forecast demand, optimize routes, and streamline inventory management, reducing costs and improving delivery times.
Key Benefits of AI and ML for Businesses
Benefit | Description |
---|---|
Improved Decision Making | AI analyzes large datasets, providing actionable insights that help businesses make informed decisions quickly. |
Operational Efficiency | Automating repetitive tasks through AI reduces operational costs and human error, leading to smoother business operations. |
Enhanced Customer Experience | AI tools personalize interactions, recommend products, and offer faster responses, improving overall customer satisfaction. |
Integrating AI into Customer Support Operations
Artificial Intelligence (AI) is revolutionizing customer support by enabling faster and more efficient service. By incorporating AI tools like chatbots, predictive analytics, and automated response systems, businesses can streamline operations and improve customer satisfaction. These technologies allow businesses to provide 24/7 support and handle a higher volume of customer inquiries without overburdening human agents.
AI integration also provides valuable insights into customer behavior and preferences, helping businesses tailor their support strategies. By analyzing large volumes of data, AI can identify common issues, predict customer needs, and personalize interactions. The result is a more responsive and effective customer service operation.
Key AI Tools in Customer Service
- Chatbots: Automate responses to common customer queries, improving response time and reducing human intervention.
- Predictive Analytics: Anticipate customer issues based on historical data and trends, allowing businesses to proactively address concerns.
- Sentiment Analysis: Evaluate customer feedback to gauge satisfaction and adjust service strategies accordingly.
Steps for Implementing AI in Support Systems
- Assess Customer Needs: Identify the most common customer inquiries and pain points.
- Select AI Tools: Choose AI technologies that align with customer service goals (e.g., chatbots, automated systems).
- Integrate with Existing Systems: Ensure smooth compatibility with current CRM tools and communication platforms.
- Train AI Models: Continuously refine AI algorithms based on feedback and performance data.
- Monitor and Optimize: Regularly review AI performance and make adjustments for optimal results.
Impact on Customer Experience
"AI integration can reduce customer wait times by up to 50%, significantly enhancing overall satisfaction and loyalty."
AI Tool | Benefits |
---|---|
Chatbots | Instant responses, 24/7 support, reduces manual workload |
Predictive Analytics | Proactive issue resolution, personalized support |
Sentiment Analysis | Real-time feedback, improved service quality |
Leveraging Machine Learning for Sales Forecasting
Machine learning is increasingly used in sales forecasting to provide businesses with more accurate predictions and insights. By analyzing historical data and identifying patterns, machine learning algorithms can predict future sales trends, helping businesses make data-driven decisions. This approach minimizes human error and allows for the optimization of sales strategies based on real-time data.
Predictive analytics powered by machine learning offers a way to enhance sales operations by forecasting demand, detecting market shifts, and improving customer targeting. The ability to predict sales outcomes allows sales teams to focus their efforts on high-potential opportunities, streamline their processes, and reduce unnecessary costs.
Key Benefits of Using Machine Learning in Sales Prediction
- Improved Accuracy: Machine learning models can process vast amounts of data and provide predictions with a high degree of accuracy, improving upon traditional methods.
- Better Resource Allocation: Predicting sales volumes helps businesses allocate resources efficiently, ensuring that inventory, staffing, and marketing efforts are aligned with demand.
- Enhanced Customer Insights: Machine learning identifies customer behaviors and preferences, allowing for more personalized sales approaches.
How Machine Learning Algorithms Work in Sales Forecasting
- Data Collection: Historical sales data, customer information, and market trends are gathered from various sources.
- Data Preprocessing: The collected data is cleaned and organized to ensure quality inputs for the machine learning model.
- Model Training: A machine learning algorithm is trained using the prepared data, where it learns to identify patterns and correlations.
- Prediction: The trained model is then used to forecast future sales, providing actionable insights for business strategies.
Example: Sales Prediction Table
Month | Predicted Sales | Actual Sales | Deviation |
---|---|---|---|
January | $200,000 | $210,000 | +5% |
February | $250,000 | $240,000 | -4% |
March | $300,000 | $320,000 | +7% |
Using machine learning for sales prediction enables businesses to anticipate demand fluctuations, optimize inventory levels, and personalize customer engagement strategies, leading to improved business outcomes.
Automating Supply Chain Management with AI Solutions
AI technologies are revolutionizing the way businesses manage their supply chains. By implementing machine learning algorithms, businesses can optimize inventory management, reduce lead times, and enhance demand forecasting accuracy. This not only minimizes operational costs but also improves the overall efficiency of supply chain operations.
One of the key benefits of using AI in supply chain management is its ability to automate repetitive tasks, providing a more agile and responsive system. AI models can predict disruptions, recommend corrective actions, and adapt to changing market conditions, allowing companies to stay ahead of potential challenges.
Key Applications of AI in Supply Chain Automation
- Demand Forecasting: Machine learning models analyze historical data to predict future demand, reducing overstock and stockouts.
- Inventory Management: AI can automatically adjust inventory levels based on real-time data, ensuring optimal stock levels at all times.
- Logistics Optimization: AI-driven systems can calculate the most efficient shipping routes, minimizing transportation costs and delivery times.
- Supplier Selection: AI can assess supplier performance and suggest the best options based on various factors like cost, reliability, and lead time.
"AI has the potential to transform supply chain operations by offering real-time data insights and predictive capabilities, ensuring businesses are more resilient to disruptions."
Benefits of Implementing AI in Supply Chain Management
- Improved decision-making through data-driven insights.
- Enhanced flexibility and adaptability in supply chain processes.
- Cost reduction through better resource utilization and reduced waste.
- Faster response times to market changes and supply chain disruptions.
Comparison of AI vs. Traditional Supply Chain Approaches
Aspect | AI-Driven Approach | Traditional Approach |
---|---|---|
Data Analysis | Real-time, predictive, automated | Manual, reactive, slower |
Inventory Control | Dynamic and optimized based on data | Fixed, based on estimates and forecasts |
Cost Management | Cost-efficient, minimal waste | Higher operational costs, wasteful processes |
Response to Disruptions | Proactive, real-time adjustments | Reactive, slower response |
Improving Marketing Campaigns with AI-Powered Insights
In today’s digital landscape, businesses are increasingly turning to artificial intelligence (AI) to optimize their marketing strategies. AI's ability to analyze vast amounts of consumer data quickly enables companies to gain valuable insights into customer preferences, behaviors, and trends. This allows for more precise targeting, personalized content, and efficient resource allocation in marketing campaigns. AI-driven tools such as predictive analytics and customer segmentation have proven to be key in enhancing marketing efforts, ensuring that businesses stay competitive and relevant in an ever-changing market.
One of the primary advantages of using AI in marketing is its capacity to deliver real-time, data-backed insights. By leveraging machine learning algorithms, businesses can refine their strategies on the fly, responding to shifts in consumer behavior almost instantly. This dynamic approach provides a significant edge over traditional methods, where adjustments often take longer and are based on limited data points.
AI-Driven Marketing Applications
- Predictive Analytics: AI models forecast future customer behavior by analyzing historical data, helping marketers anticipate trends and align campaigns accordingly.
- Personalization: AI tools create tailored experiences for users, recommending products or services based on individual preferences and browsing history.
- Customer Segmentation: AI clusters customers into distinct groups, allowing businesses to create targeted, customized messaging that resonates with each segment.
- Content Optimization: Machine learning algorithms help identify the best-performing content, ensuring that marketing materials are aligned with audience interests.
Key Benefits of AI in Marketing
Benefit | Description |
---|---|
Efficiency | AI reduces manual work by automating tasks like data analysis, customer interactions, and content recommendations. |
Improved Targeting | AI enables more precise targeting of ads, emails, and promotions, increasing the likelihood of conversion. |
Cost-Effectiveness | By optimizing resources and improving decision-making, AI minimizes wasted spend in marketing campaigns. |
AI enables marketers to create campaigns that not only reach the right audience but also deliver content that resonates deeply, enhancing customer loyalty and driving business growth.
Leveraging Machine Learning for Fraud Detection in Financial Transactions
In today's financial landscape, the volume of transactions has increased exponentially, making it more challenging to manually detect fraudulent activities. Machine learning (ML) offers a powerful approach to combat these issues by automating and enhancing fraud detection systems. By utilizing algorithms that can recognize patterns and anomalies in transaction data, businesses can identify suspicious activities in real-time, minimizing potential losses.
ML models can analyze vast amounts of transaction data, including purchase histories, geographic locations, and time patterns, to flag transactions that deviate from a customer's usual behavior. The ability of these models to continuously learn from new data ensures that they adapt to evolving fraud tactics, enhancing the accuracy of detection and reducing false positives.
Key Machine Learning Techniques in Fraud Detection
- Supervised Learning: This approach involves training the model on a labeled dataset that includes both legitimate and fraudulent transactions. The algorithm learns to differentiate between the two based on features such as transaction amount, frequency, and location.
- Unsupervised Learning: Unsupervised models identify anomalies in transaction patterns without the need for labeled data. These algorithms are useful when historical fraud data is scarce or unavailable.
- Deep Learning: Deep neural networks can analyze complex data structures, including images or text, making them ideal for detecting sophisticated fraud techniques such as identity theft or synthetic fraud.
Machine learning allows for continuous learning and improvement of fraud detection models, adapting to emerging threats and new patterns of fraud.
Fraud Detection Workflow
Step | Action |
---|---|
1 | Data Collection: Collect relevant transaction data such as customer behavior, transaction details, and historical fraud patterns. |
2 | Data Preprocessing: Clean and structure the data for model training, including handling missing values and normalizing features. |
3 | Model Training: Train the machine learning model using appropriate algorithms, such as supervised or unsupervised learning. |
4 | Real-time Prediction: Implement the trained model in a live environment to evaluate transactions and flag potentially fraudulent ones. |
5 | Continuous Monitoring: Continuously monitor and retrain the model with new data to ensure its accuracy and adapt to new fraud tactics. |
Leveraging AI to Tailor User Experience in Online Retail
AI-powered personalization is revolutionizing the e-commerce industry by enabling businesses to create highly customized experiences for their users. By analyzing customer behavior, preferences, and purchasing patterns, AI can deliver product recommendations, dynamic pricing, and targeted marketing strategies that align with individual needs.
As consumers increasingly expect a personalized shopping journey, companies are turning to AI-driven tools to stay competitive. This technology not only enhances user satisfaction but also boosts sales and customer loyalty by presenting the right products at the right time, improving customer retention rates.
AI Techniques for Personalization
- Recommendation Engines: AI algorithms analyze user behavior and product history to suggest items that match their tastes.
- Dynamic Pricing: AI adjusts product prices in real-time based on market conditions, customer demand, and competitor pricing.
- Personalized Search Results: By understanding the user’s browsing history, AI can prioritize search results based on preferences and past interactions.
Benefits of Personalization
"Personalization powered by AI not only improves user experience but also drives higher conversion rates and customer loyalty."
- Improved Conversion Rates: Personalized recommendations lead to higher likelihood of purchases, increasing sales opportunities.
- Enhanced Customer Loyalty: Offering a tailored shopping experience helps foster stronger relationships with customers, encouraging repeat business.
- Cost Efficiency: AI minimizes the risk of overstocking and understocking by predicting customer demand more accurately, optimizing inventory management.
Example of AI-Driven Personalization
AI Tool | Application | Result |
---|---|---|
Collaborative Filtering | Recommends products based on similarities to other users’ behavior | Increased sales by suggesting items users are likely to buy |
Content-Based Filtering | Recommends products based on attributes like style, size, or color preferences | Higher customer satisfaction as products match their preferences |
Predictive Analytics | Predicts future purchase behavior to deliver tailored promotions | Boosted conversion rates and higher return on investment (ROI) |
Streamlining HR Processes Through AI and Machine Learning
Artificial Intelligence (AI) and Machine Learning (ML) are increasingly playing a crucial role in optimizing HR workflows. By automating repetitive tasks and providing data-driven insights, organizations can enhance their HR operations. These technologies allow HR departments to manage employee data more efficiently, improve recruitment processes, and ensure better employee retention through predictive analytics.
AI and ML applications in HR processes go beyond just administrative tasks. These tools are helping HR professionals make smarter, faster decisions based on vast amounts of data. From recruitment to employee performance management, here’s how AI and ML are transforming human resources:
Key HR Areas Improved by AI and ML
- Recruitment and Hiring: Automating candidate screening and matching resumes with job descriptions.
- Employee Retention: Predicting potential turnover and offering retention strategies.
- Performance Management: Analyzing employee performance data and suggesting development opportunities.
- Training and Development: Personalizing employee training based on learning patterns.
Examples of AI Tools in HR
- Chatbots for Candidate Interaction: AI-driven chatbots can answer applicant queries, schedule interviews, and provide updates.
- Predictive Analytics for Employee Turnover: ML models that predict turnover risk based on factors like work satisfaction, tenure, and job role.
- Automated Performance Reviews: AI analyzes performance data and provides unbiased feedback, eliminating human errors in the review process.
Benefits of AI and ML in HR
Benefit | Description |
---|---|
Efficiency | Automates repetitive tasks like resume screening and administrative work, freeing up time for HR professionals. |
Data-Driven Decisions | Provides insights into employee performance, satisfaction, and potential risk, leading to informed decision-making. |
Cost Reduction | Reduces the cost of manual tasks and minimizes human errors that can result in costly mistakes. |
AI and ML are not just tools for automation but also for predicting trends and identifying patterns that can help HR make proactive decisions.